CPHA Collaborator Panel Session: Exploring the Ethics of AI Approaches in Public Health
Background (Purpose and Objectives)
The Canadian Institutes of Health Research’s Institute of Population and Public Health (CIHR-IPPH) hosted a Session at Public Health 2018 in collaboration with the Canadian Institute for Advanced Research (CIFAR) on May 29, 2018 in Montreal, to discuss ethical challenges related to integrating Artificial Intelligence (AI) approaches into public health research and practice.
The objectives of this session were to:
- Increase awareness of ethical challenges related to public health that are posed by AI approaches
- Develop greater understanding of potential impacts of AI on health equity
- Identify potential roles for public health practitioners, policymakers, ethicists, researchers, and private/innovation stakeholders to address ethical challenges in AI
The expert panellists invited to lead the discussion were:
- Dr. David Buckeridge - Professor of Epidemiology and Biostatistics, McGill University (Public Health Research)
- Ms. Erin Kelly - CEO, Advanced Symbolics (Private/Innovation Sector)
- Dr. Joelle Pineau - Professor School of Computer Science, McGill University; Senior Fellow, CIFAR Learning in Machines & Brains Program (Artificial Intelligence Research)
- Dr. Denis A. Roy – Vice President of Science and Clinical Governance, INESSS (Institut national d’excellence en santé et en services sociaux / Institute for Excellence in Health and Social Services) (Policy/Implementation)
- Dr. Bryn Williams-Jones - Professor and Director of Bioethics, Université de Montréal (Ethics)
Key Messages from Panel Discussion
FAT ML: Dr. Pineau noted the value of ML as transformative for areas where there is uniform data, and that the challenge lies with using ML technology to analyze rich, heterogenous data, which is frequently used in public health research.
Figure 1 - Artificial Intelligence (AI) - as defined by Dr. Joelle Pineau
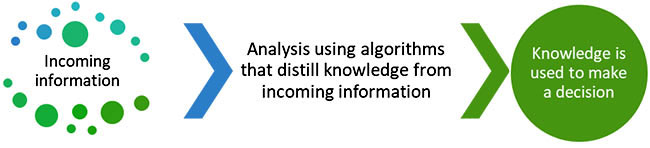
Long Description
Step 1: information is gathered and compiled
Step 2: analysis using algorithms then distils knowledge from incoming information
Step 3: generated knowledge is used to make a decision.
Due to this complexity of public health data, the main ethical concerns with respect to public health can be coined by the term “FAT ML”, which refers to fairness, accountability, and transparency of machine learning, and is a fundamental concept for both theoretical and applied uses of AI.
- Fairness: how do we guarantee that the machines making decisions are making ones that are impartial, and are just, particularly for those that are living with those decisions? Fairness should be considered in how we design algorithms, how the data is encoded, and fundamentally who collects the data.
- Accountability: How do we decide to attribute responsibility for cases where decisions were made by systems? The notion of accepting responsibility is not the same for machines – is it sufficient for systems to do this?
- Transparency: We want to have a system that can explain its behaviours, but machines are less equipped to explain these decisions.
Honest, Critical and Open Debate: Dr. Bryn Williams-Jones introduced ethics into the discussion by noting that the social and policy debate regarding AI stokes fears about privacy, exclusion and potential disadvantages to some groups over others. Science takes a long time, and the way we sell science, including promising the potential of its products, affects public policy. How do we balance these pressures to deliver as stakeholders, given the social structures of how science and policymaking occur? Dr. Williams-Jones argued that the way to address the challenge to meet promises of science in policymaking is to have honest, open and critical debate about who is socially responsible for AI, for what are they responsible, and who is building these technologies. The challenge for public health is having honest communications about what we don’t know. Science is fundamentally socially embedded, and therefore, it is critical to engage both scientists and the general public in honest discussions about what we do and do not know about AI relevant to public health.
Opportunity Cost for Public Health: Dr. Buckeridge highlighted that surveillance in public health has evolved, and that we need sophisticated data and analysis methods exploring the causal frameworks that drive health conditions and inequalities we see in society, such as precision public health which weighs societal benefits and individual risks. Public health is not embracing the use of new technologies to analyze detailed individual-level data in order to understand driving factors leading to public health conditions and how to effectively respond to these issues. He proposed that the lack of uptake is attributed to limited resources, training and capacity to support understanding data and resulting analyses from using AI tools. There is a clear opportunity cost that public health is taking by not making data available for public health organizations to analyze with AI tools, and this could lead to an imbalance in understanding benefits of these AI tools for society.
Transparency in AI Methodologies: Ms. Kelly stressed that with larger data sets, there are opportunities to collect data that are more representative of populations across Canada. To act on complex challenges such as suicide prevention and the opioid crisis, where populations are often hard to reach – AI technologies and using social media may make it easier to reach these populations than using traditional public health methods.
However, Ms. Kelly argued that organizations using these technologies need to be open to walking knowledge users through the methodology they use, and public health organizations and researchers working with industry organizations have to understand the methodologies being used – otherwise, how does one ensure if these are repeatable in other contexts or truly representative? There are so many AI tools out there to use, but not many open, transparent methodologies that organizations are willing to share. Public health researchers and organizations using these AI tools and the end clients affected by the results should be aware, and should actively ask for mechanisms and algorithms developed to generate knowledge.
Unrealized Value, Collective Governance and Citizen Leadership: Dr. Denis Roy emphasized that the unrealized value of data and information in the way that public health does business is an ethical challenge, leading to inefficient use of resources. He characterized the political challenge of not realizing the value of AI tools to analyze public health data as an omission, as “we are not doing what we should be doing.” Revisiting ethical rules of using AI for research in health systems will enable public health decision-making to better apply the use of AI technologies to generate evidence that supports health systems policy and implementation. Dr. Roy argued that we need strong citizen leadership and new collective governance, with legitimacy being accomplished if we have citizens and leadership capacity committed to the common good for public health. The open source domain is a key area of interest which needs to be protected, but publicly available and transparent so that populations can understand who is doing what with their data, and how it will be used to support population health and wellness.
Summary: Moderated Discussion with Panel and Session Participants
The following key points and suggestions were made in response to the panel talks from Dr. Joelle Pineau, Dr. Bryn Williams-Jones, Dr. David Buckeridge, Ms. Erin Kelly, and Dr. Denis Roy and group discussion with session participants in-person and on Twitter, moderated by Dr. Steven Hoffman.
Explainability: It can be difficult at this point to get AI tools to explain why they arrive at the conclusions that they make, but in the meantime, we can ensure that the methodology is transparent, and examine what are the training datasets used to prime those AI tools.
Capacity-building for Action: We have to rethink our decisions to improve education for public health stakeholders in using and acting with knowledge derived from AI tools. We can do this if there are partnerships established between technical scientists, such as computer scientists and engineers, and people utilizing decisions or interpreting knowledge generated using AI tools, such as public health researchers, practitioners, and ethicists. Further dialogue is needed to determine how to use AI to help us act in public health.
Accountability and Governance: An emerging issue for public health decision-making is that new information continues to be generated, but we are not taking advantage of understanding and developing actions based on this information. It is important to question our accountability from a health system governance perspective – if AI tools are more precisely predicting situations in public health such as epidemics or the effects of cannabis legalization on specific communities, who is accountable to act on this information? Therefore, predictive analytics mobilized by AI tools is essential to consider as a new dimension for public health practice and decision-making.
Autonomy Levels of AI Systems in Public Health: There are four levels which highlight increasing autonomy and decreasing human involvement of AI systems taking action, and in health we are largely still operating at the first two levels (Figure 2):
- enhancing situational awareness using AI,
- prediction;
- AI systems are making recommendations, beyond prediction, on courses of action; and
- AI systems conducting interventions directly.
Figure 2: Levels of Autonomy of AI Systems – as defined by Dr. Joelle Pineau
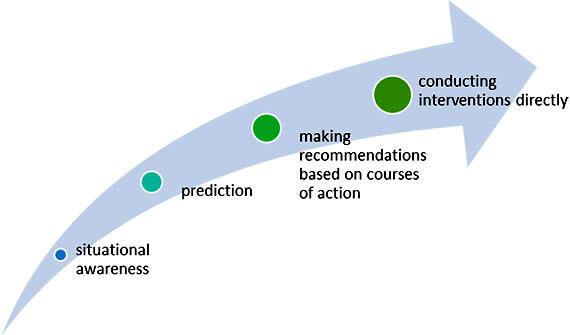
Long Description
- situational awareness
- prediction
- making recommendations based on courses of action
- conducting interventions directly
For each of these levels, careful consideration will have to be given to how the application of AI may impact public health decisions and outcomes.
Privacy and Data-sharing Concerns: A participant asked what governments can do to accommodate the balance needed for public health to use AI tools while also ensuring privacy of data, highlighting the health sector’s privacy and data-sharing concerns and the existing “chicken-and-egg” situation between the use of AI tools and ethics surrounding them. Panelists suggested there are sectors and organizations that are risk-averse, but there are benefits and the government is receptive to acting on using AI tools for current public health issues such as the opioid crisis. Framing effects can also be used as a model – by having stakeholders reframing the issue from a protectionist approach to a prevention approach arises the opportunity to identify common ways to address existing challenges.
Principles of Justice in the Use of AI: A participant online posed a Twitter question about the principles of justice with respect to AI use in surveillance and data collection, and this prompted the panel asked participants to unpack the term “justice” – are we seeking social justice, or distributive justice? The way we make decisions in public health depends on what kind of justice in particular we seek to achieve.
Public Health Position Statement on AI and Big Data: Panelists agreed that both the CPHA, and public health as a sector, have a role to articulate the message that there are clear costs for not sharing data that could save lives. Data is a social determinant of health, and it is up to public health to determine how it can be better used. Examples of existing initiatives mentioned include the Farr Institute’s #DataSavesLives, and the Open Data Movement. However, finding the balance between rich and complex data, and analyzing it can be difficult, and it is important to articulate principles for which public health makes decisions and the potential risks to privacy to maintain public good. There is an ethical responsibility to share methods so that data can be analyzed across sites simultaneously and in public health practice, and public health has a strategic advantage to encourage collaboration across settings.
Mobilizing AI Champions: Panelists suggested that it is easier to integrate champions of AI into respective public health organizations, as this will activate more change than top-down decision-making. Funding organizations, such as CIHR, have a critical role in coordinating and funding avenues for discussion, research, and activities that will help to build capacity, empower researchers and advocates to work in this space, and support the growth of networks.
Ensuring Fairness: Panelists argued that fairness begins from the point of origin of the data, starting off with who is collecting the data. There are technical ways of building variables into algorithms, in order to explore biases, as well as build and train algorithms to predict a particular factor, but there also needs to be consideration of what kinds of data are generated in our society, and how it is being generated. Panelists also reinforced that we should continue to strive for representative sampling of populations to ensure that we actively work to be inclusive, and adopting responsible strategies to sample from traditionally “hard to reach” populations.
Job Security: It is likely that we will see a huge transformation in the types of jobs that are available with AI being further incorporated into the job market. Panelists noted that AI researchers are not as afraid of AI taking over jobs compared to what people hear in the media, referencing the example of airplanes having autopilot ability, but still requiring pilots for safety purposes. However, real concerns with autonomous buses and other technologies exist, and the panelists suggested that jobs will change while requiring customer service and safety measures which will need human intelligence.
Balancing Use of AI in Public Health: A session participant asked the panel on Twitter how can public health balance its use of AI in a world where there are actors using AI who do not share the same values that guide public health. Panelists encouraged participants to think about how the public health community engages with stakeholders and communities in other sectors, and offered that we should articulate our basic principles with these communities. Pushing for transparency from all stakeholders could be a net win for public health, and identifying what is tolerable risk and how to balance this with benefits for the common good could be a constructive public health and AI initiative.
Final Reflections
Dr. Steven Hoffman summarized key takeaways from the session, and called on participants to continue to engage in the discussion, including on Twitter using the hashtag #publichealthAI.
Key Takeaways from the discussion included:
- Existing common thread of transparency and explainability for data used, the methods, and the application of the results
- Public health is implicated by considerations for FAT ML
- Accessibility of data and issues around privacy are key challenges
- Accountability and governance were identified as issues particularly relevant to health systems – both for who is making decisions and who is accountable to those that are impacted by these decisions? Addressing this opportunity requires citizen leadership
- With respect to autonomy levels, AI systems in public health are currently situated between making predictions and making recommendations on courses of action
- Open engagement and dialogue with the public and reframing the conversation from a prevention approach is needed.
- Using collective commitment to common good as way to connect with other sectors
- Date modified: